Secure and Trustworthy ML 2024: A home for machine learning security research
Attendees of the 2nd IEEE Secure and Trustworthy Machine Learning (SaTML) conference listen to a tutorial on detecting copyrighted documents in LLM training sets by Yves-Alexandre de Montjoye of Imperial College London. Below, engineering science student and Vector Institute research intern Sierra Wyllie summarizes the conference’s activities. All photos by Sierra Wyllie.
How can we help people recognize AI-generated images? Can we prevent copyrighted materials from being used in training data? What’s going on in the new field of forensic analysis of ML systems?
These and related topics were at the centre of the second installment of the Secure and Trustworthy Machine Learning (SaTML) conference, which took place in Toronto from April 9th to 11th, 2024 under co-sponsorship from the Schwartz Reisman Institute for Technology and Society. This year, the conference published 34 papers ranging in topics from protecting user privacy and fairness to proposing new security attacks on ML systems.
The conference included nine presentation sessions and two poster sessions dedicated to the published works, as well as keynotes, tutorials, and a competition track addressing open challenges in ML security.
Let’s explore some highlights.
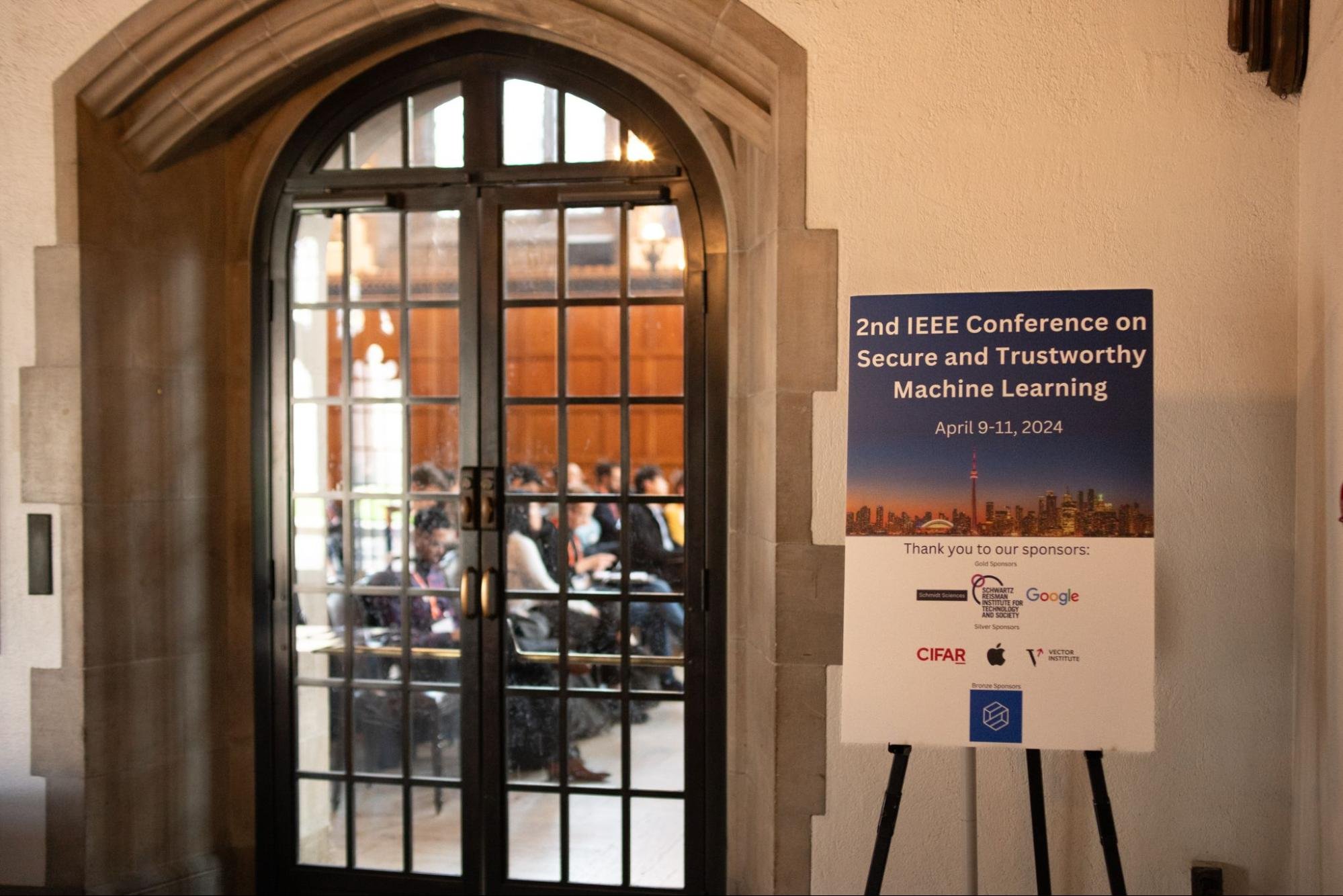
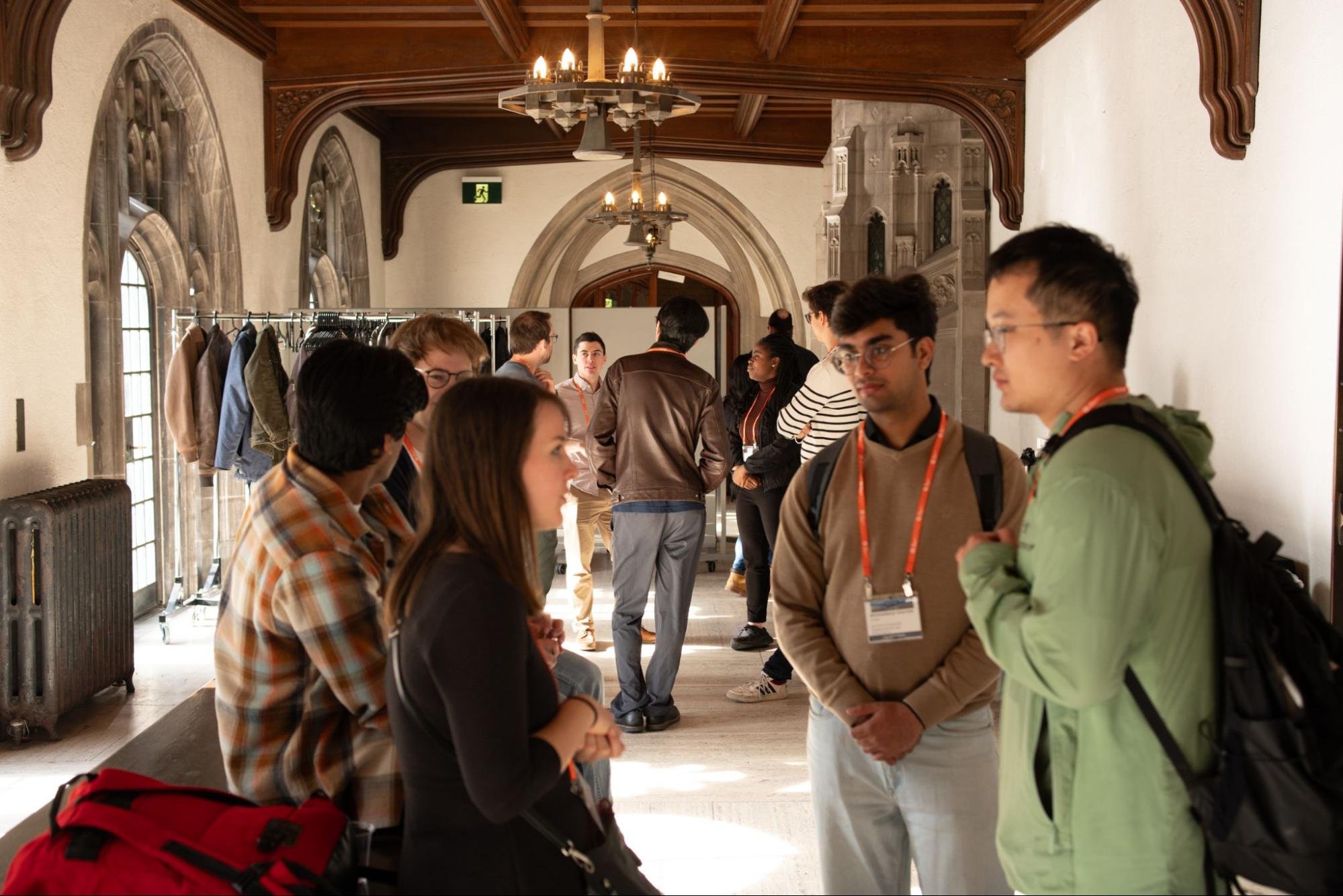
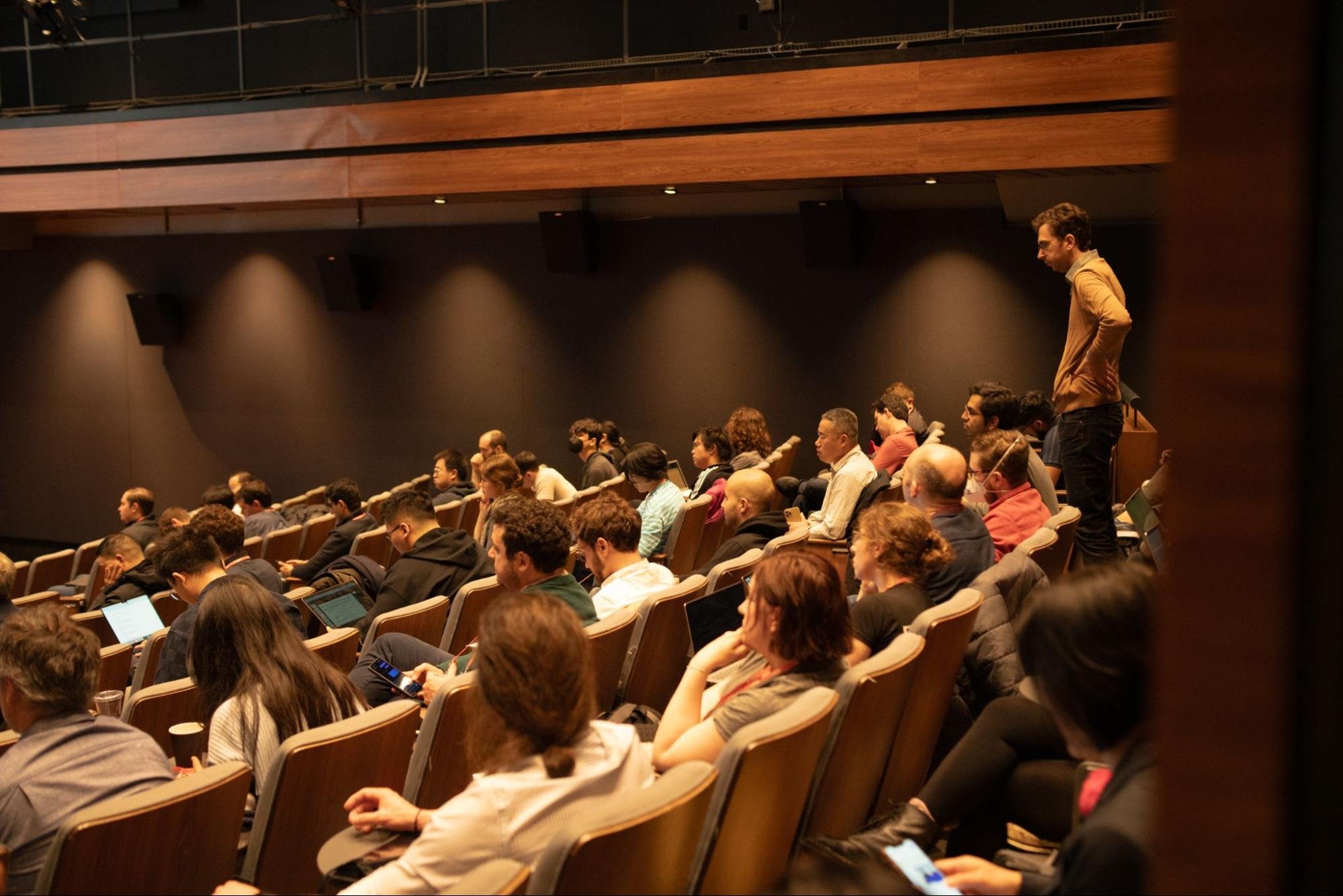
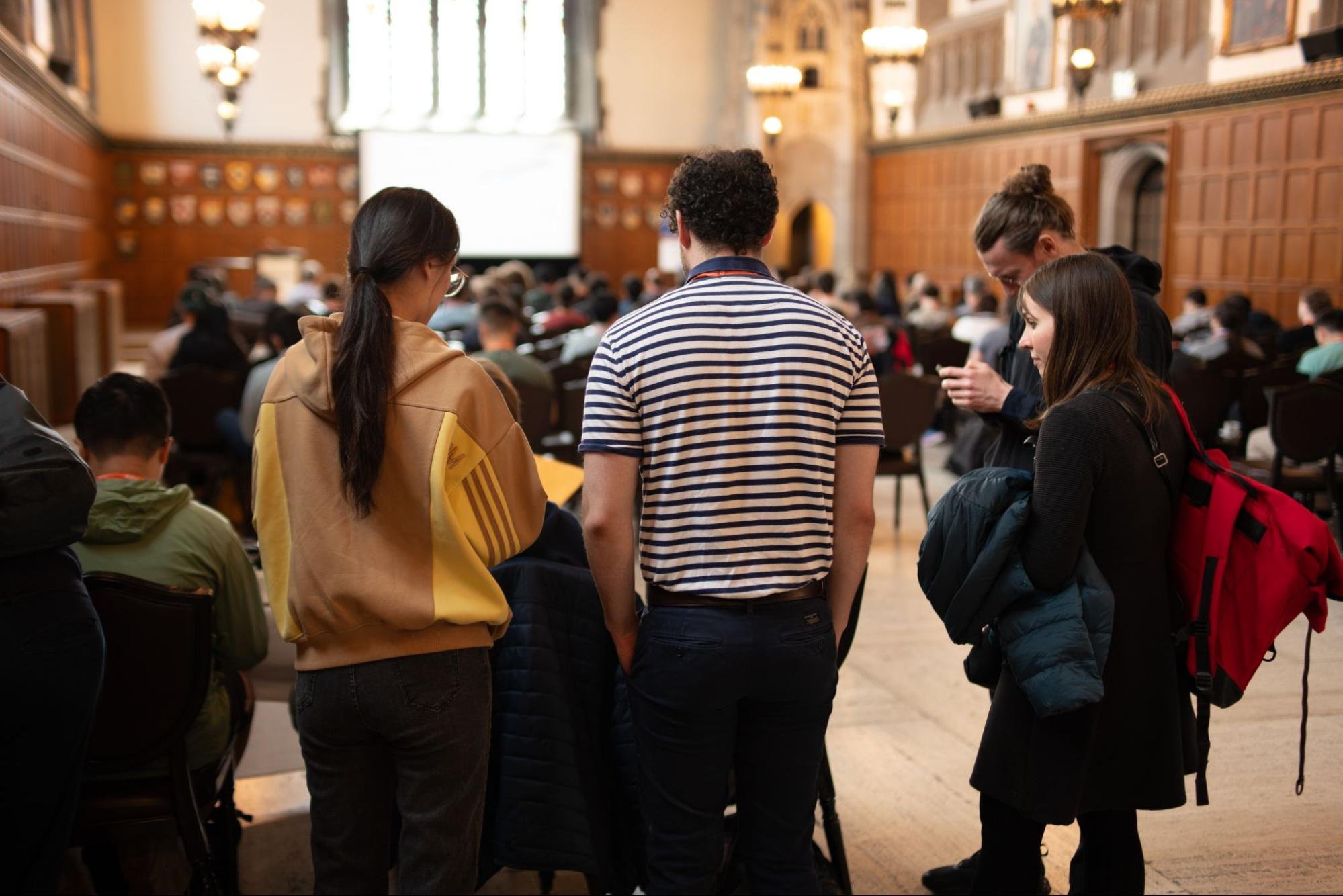
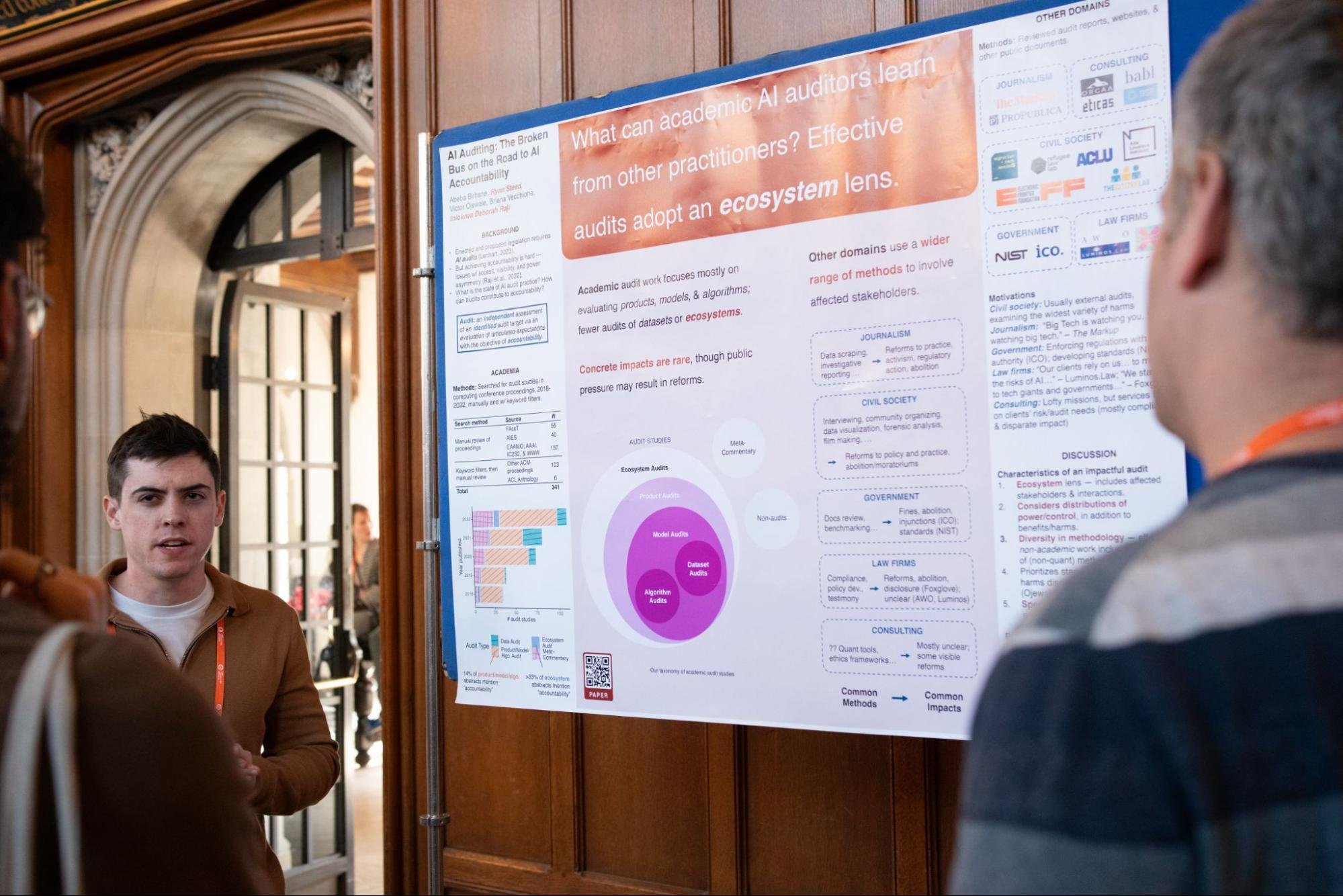
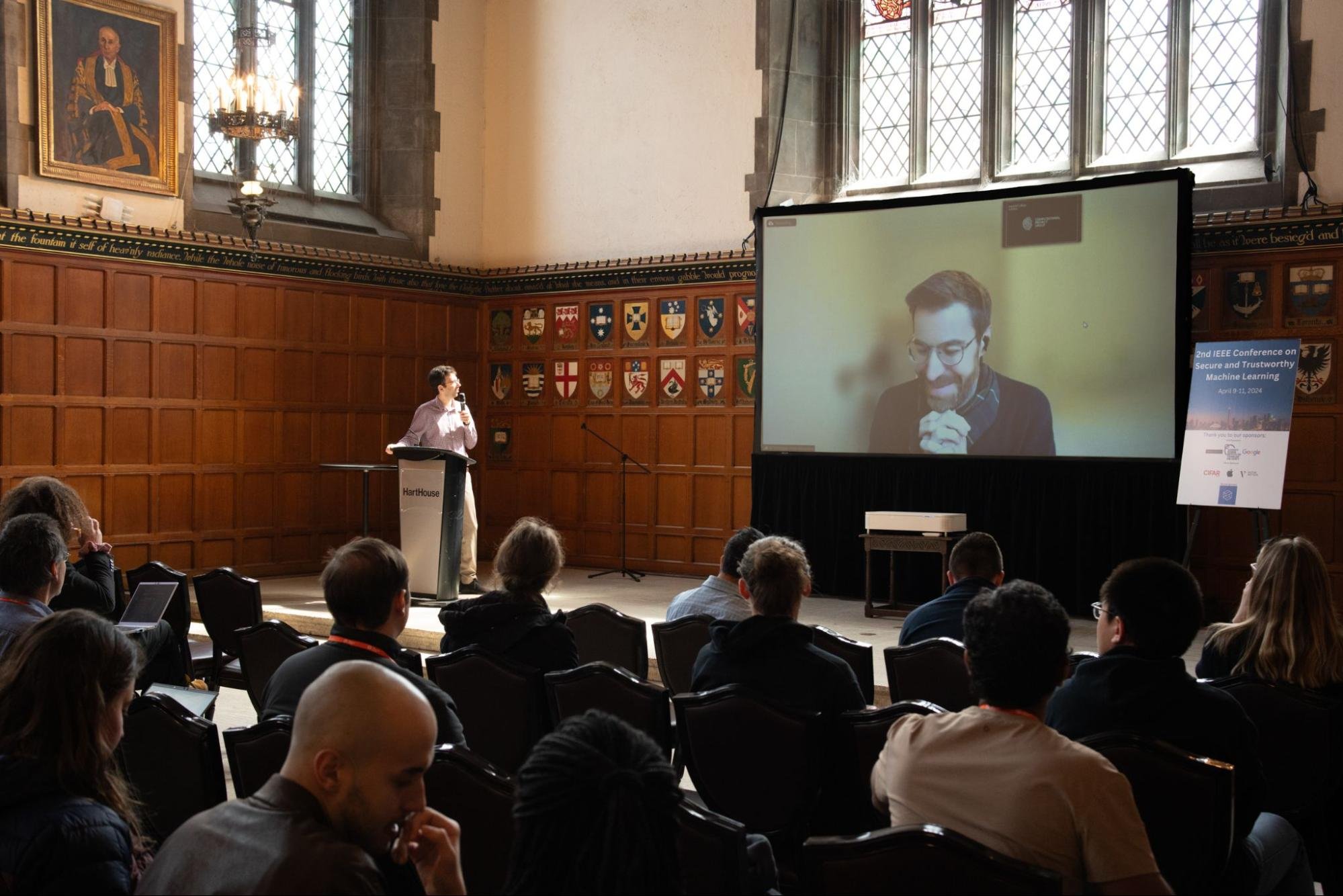
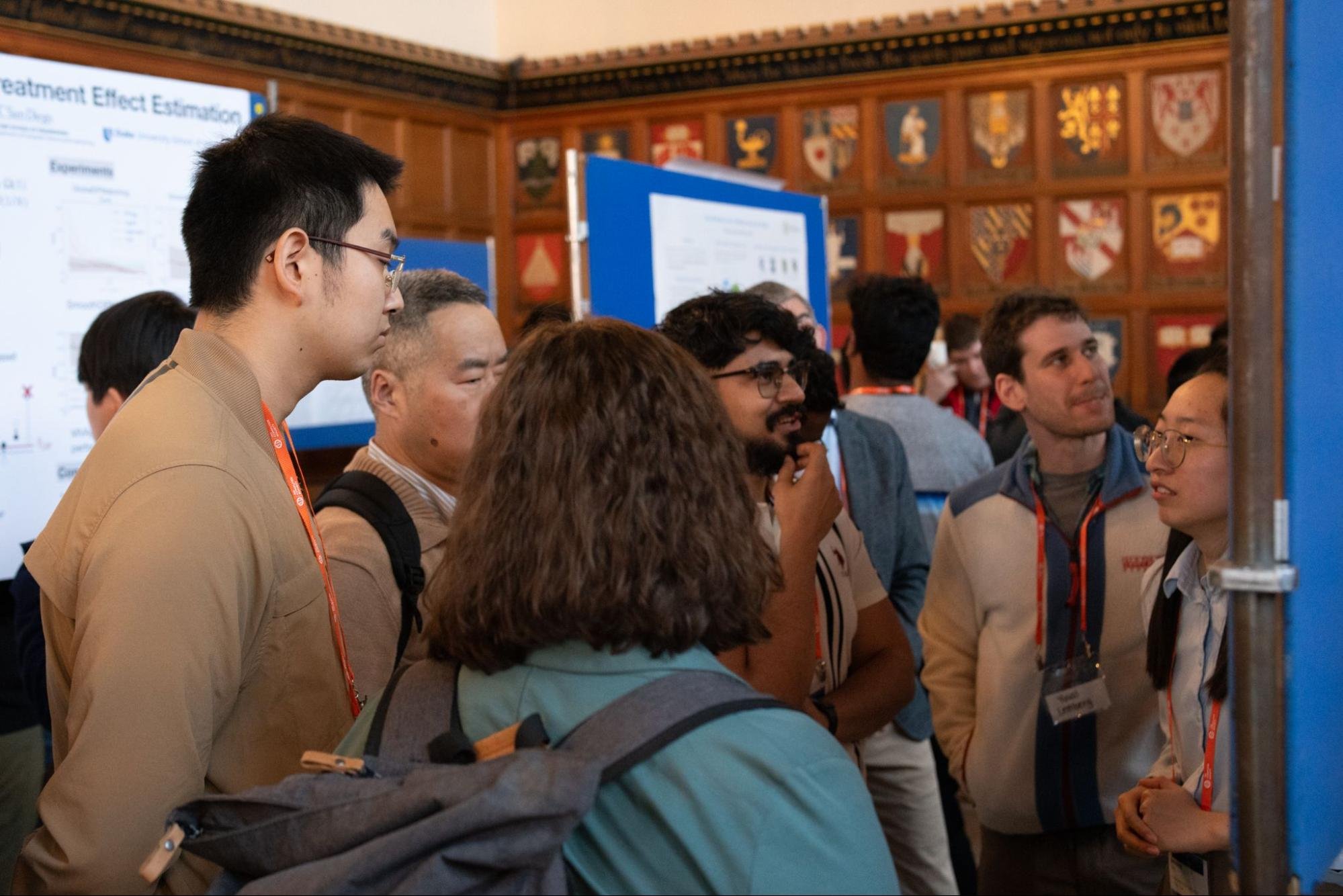
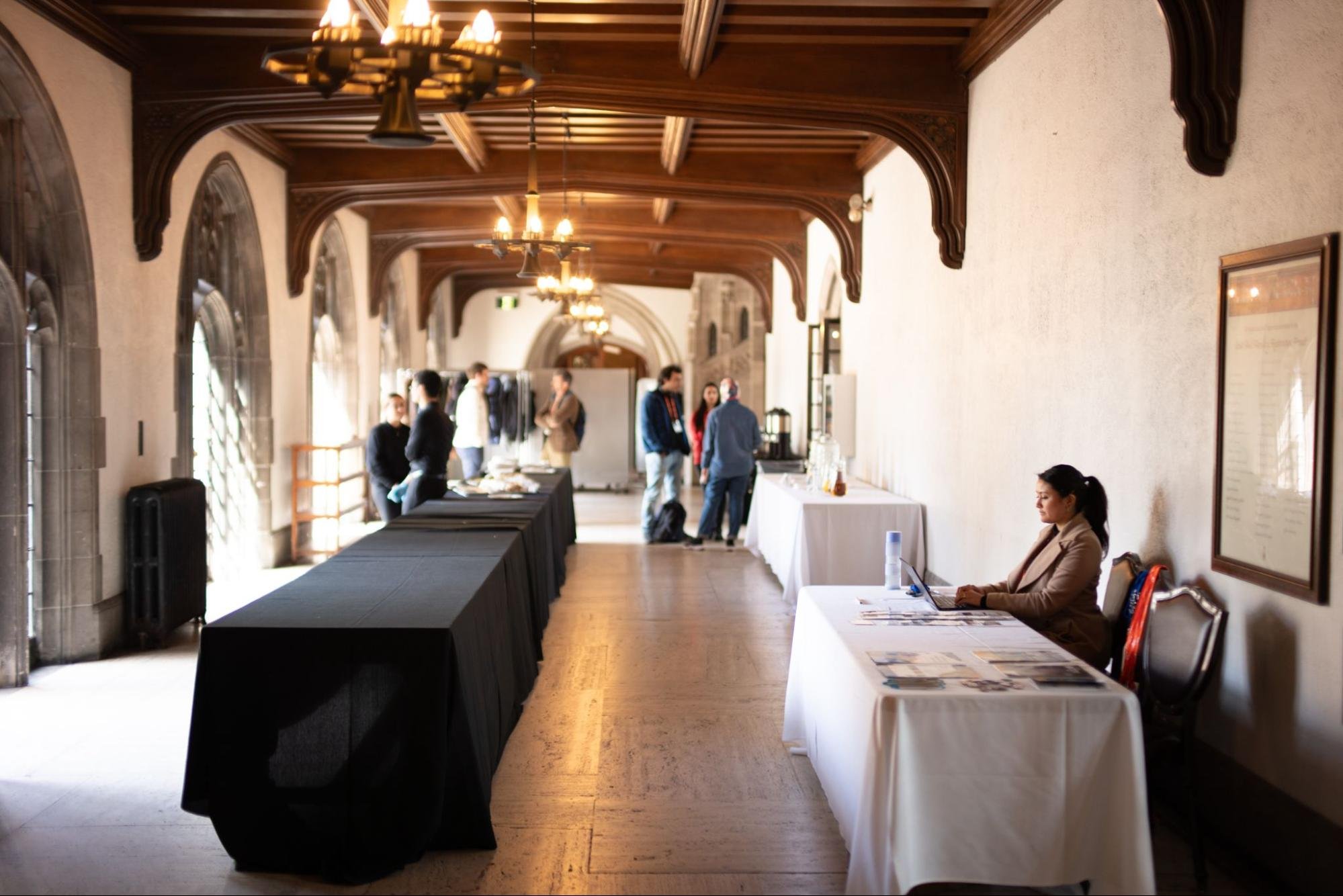
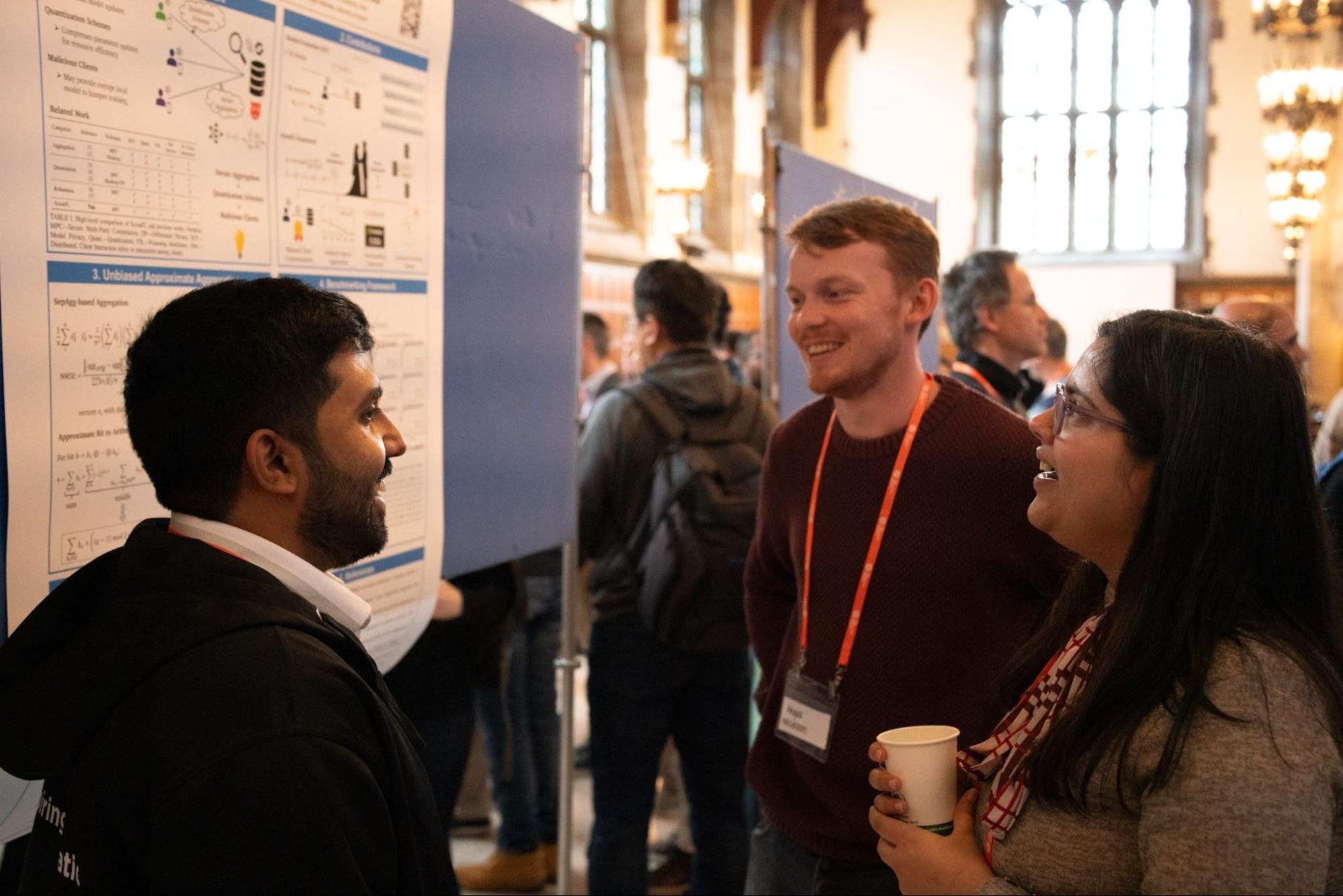
Explore a slideshow from the the 2nd IEEE Secure and Trustworthy ML conference. All photos by Sierra Wyllie.
Detecting AI-generated outputs: an impossible challenge?
On April 9th, the conference heard from its first keynote speaker, Somesh Jha, a respected computer security researcher currently working on adversarial ML. Jha’s talk focused on watermarking for AI systems that generate images or text (think ChatGPT or DALL-E). As in photography, a watermark would be displayed on the outputs of a generative AI model, informing users that the image or text was AI-generated.
Ideally, this would prevent misinformation and deception by generative AI; in fact, many national AI laws mention watermarking for this purpose. However, Jha identified several methods that can remove watermarks, thereby allowing generated data to pass as human-created.
Instead of addressing how watermarking might be made tamper-proof, Jha instead identified several alternate uses for watermarks. For example, when AI-generated data is used for training a new generative model, the new model might replicate the mistakes or biases of the older data. By filtering AI-generated data out of future training sets using watermarks, we can train models independently of past model mistakes.
Jha also identified a new concern about watermarking: “Holding my cynical hat on, I think [large AI companies] are much more worried about [watermarking] forgery. I could make a bad article about myself, embed a watermark in it, and say that it came from GPT-4. I could then probably drag [a large AI company] into a lawsuit.”
Somesh Jha gives the first keynote on the current state of watermarking for generative AI models. Jha is Lubar Professor in the Computer Sciences Department at the University of Wisconsin.
Jha’s keynote was later reflected in a tutorial by Yves-Alexandre de Montjoye, associate professor of applied mathematics and computer science at Imperial College London. De Montoye delivered a tutorial on detecting copyrighted data used in training large language models. He identified an approach called “document-level membership inference attacks,” which might be able to identify a document in the training data from its prevalence in generated text.
Detectives at work in ML: auditing and forensic analysis
Mozilla Fellow and UC Berkeley PhD student Inioluwa Deborah Raji delivers the second-day keynote on the topic of auditing models.
The second keynote was delivered by Inioluwa Deborah Raji, a Mozilla fellow, UC Berkeley PhD student, and one of the most well-known researchers today in algorithmic bias and accountability.
Raji’s keynote on the challenges of model auditing introduced the goals of algorithmic audits, which extend beyond traditional silos of machine learning research.
“The goal of audits is to get us to accountable outcomes; they’re not just evaluations” Raji said. After overviewing the institutional and legal policy landscapes for AI audits, she returned to a core motivation for the field.
“When these AI systems fail, it’s not just that they’re failing arbitrarily and causing harm arbitrarily. Those who seem to be the most impacted are those that are the most misrepresented or underrepresented in the data, or those that are disproportionately subject to these AI deployments in a way that can cause harm.”
Following Raji’s keynote was a session dedicated to forensic analysis of ML systems, a new field broadly seeking to uncover causes for model outputs, including model failures.
Model attacks and their defenses
The “secure” aspect of SaTML populated most of the third and final day. There were two sessions dedicated to model attacks and defenses: one focused on vulnerabilities when training models, and the other on using models.
The session on training-time attacks—which are attacks leveled at ML models during the time of their training, then exploited once they are deployed—primarily featured backdoor attacks. Backdoor attacks involve attackers inserting a subtle, perhaps not noticeable “backdoor”—like a secret loophole—into an ML model and/or its training data, so that when the attacker executes some mechanism for activating the backdoor, the model is compromised. One canonical attack added a small Hello Kitty logo to the corner of an image and changed its label to the backdoor label. Once the model has been trained and published, the attacker could then add the Hello Kitty trigger to the image, which would force the model to output the backdoor label.
One particularly interesting paper at SaTML identified a completely undetectable backdoor attack called ImpNet. An attacker might change just a few pixels in image models or words in language models to trigger a backdoor they created in the compiler. The compiler in computer systems is responsible for translating the code in which an ML model is written into instructions a machine, but not a human, can understand.
Paper author Eleanor Clifford, a PhD student at Cambridge University, issued a warning during her paper’s presentation: “Don’t accept a compiler that is proprietary if you can’t audit it, unless it's been audited by someone you trust.”
Eleanor Clifford, author of the ImpNet backdoor attack and PhD student at Cambridge University, discussing research with Anvith Thudi, a PhD student at the University of Toronto.
Overall, the 2024 iteration of SaTML fostered an atmosphere of collaboration and discussion, tackling the most pressing issues of ML security and trustworthiness.
“It’s been really exciting seeing the community we started putting together at workshops and machine learning and security conferences now have its own home,” said conference co-chair Nicolas Papernot, who is a faculty affiliate at the Schwartz Reisman Institute for Technology and Society as well as an assistant professor in the Department of Electrical and Computer Engineering and the Department of Computer Science at the University of Toronto. Papernot co-chaired the conference with Carmela Troncoso, associate professor at the École Polytechnique Fédérale de Lausanne in Switzerland.
Want to learn more?
About the author
Sierra Wyllie is a fourth-year student in the Engineering Science Program at the University of Toronto advised by Nicolas Papernot. Her research focuses on the fairness and security of machine learning systems and their sociotechnical embeddedness. Her most recent paper shows how training ML models on previously ML-generated data leads to increases in the unfairness of models. She is a recipient of the Natural Sciences and Engineering Research Council of Canada undergraduate student research award and a national honorable mention of the National Center for Women in IT in the U.S. Currently, she is co-organizing the first Toronto Ethics in AI Symposium to build a community of students interested in AI ethics at universities around Toronto. In addition to her academic pursuits, she is also an amateur photographer.