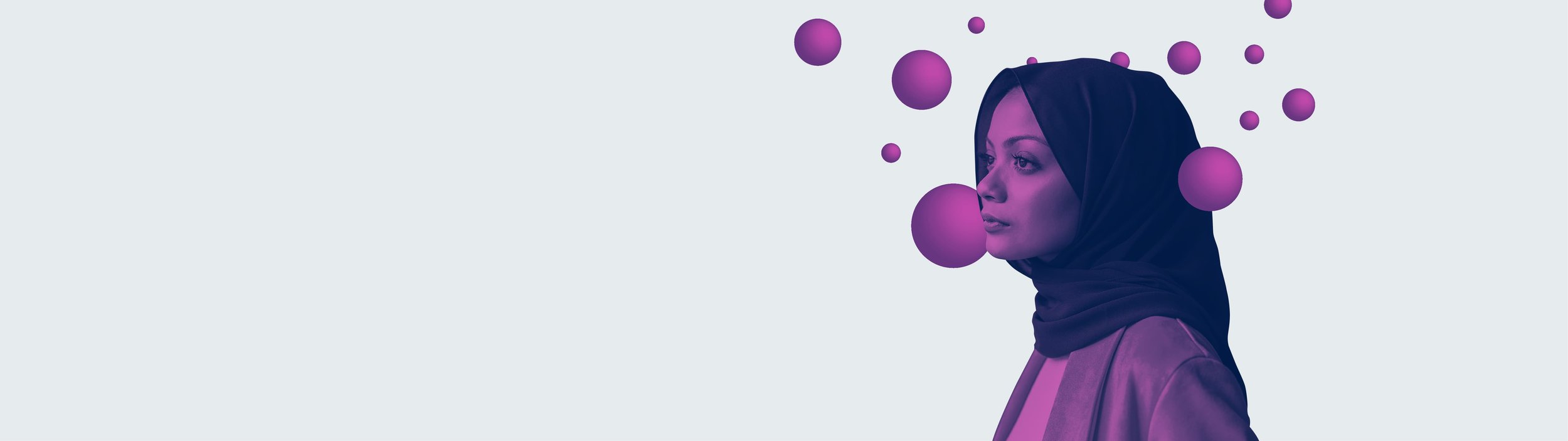
Videos
Browse the videos below to see what we’ve been thinking about and working on.
Absolutely Interdisciplinary 2022
An annual academic conference hosted by the Schwartz Reisman Institute for Technology and Society, Absolutely Interdisciplinary convenes leading thinkers from a rich variety of fields to engage in conversations that encourage innovation and inspire new insights. Connecting technical researchers, social scientists, and humanists, Absolutely Interdisciplinary fosters new ways of thinking about the challenges presented by artificial intelligence and other powerful data-driven technologies to build a future that promotes human well-being—for everyone.
Conference participants will contribute to and learn about emerging research areas and new questions to explore. Each session pairs researchers from different disciplines to address a common question and facilitate a group discussion. By identifying people working on similar questions from different perspectives, we will foster conversations that develop the interdisciplinary approaches and research questions needed to understand how AI can be made to align with human values.
Redrawing data boundaries
Companies have many incentives to draw fences and boundaries around the data they collect and process, whether for use in training AI models or other purposes. These boundaries can be legally constructed with a variety of tools, including intellectual property rights, privacy rights, and contracts. Should we instead be developing rights of public access to this data? Or recognize that some of this data should be a public resource? When this data is data about persons how can we also respect the interests of data subjects?
Speakers: Lisa Austin (moderator), Eric Horvitz, Aziz Z. Huq, Robert Seamans, Pamela Snively
Explanation and justification in AI
Modern machine learning systems are often large and complex, making it difficult to understand why they do what they do. This “black box” problem raises challenges when AI systems are used to make or contribute to important decisions such as what medical treatments to adopt, whether to grant bail to someone pending criminal trial, or how to distribute public benefits. The call for AI to be “explainable” has thus been mounting for several years and a “right to explanation” is beginning to appear in proposed legislation governing AI. This call has spurred computer scientists to develop methods to provide accounts of what factors produce or influence an AI decision. But are such accounts the only kinds of explanations we need if AI is to play a significant role in our societies? Do we need explanation, or do we need justification? We look for justification from decision makers such as judges and regulators—an account of the reasons that show that a decision is consistent with governing rules and principles. What insights might we gain about how to build trustworthy and accountable systems from what we know about justification in legal and regulatory domains?
Speakers: Boris Babic, Philippe-André Rodriguez (moderator), Finale Doshi-Velez
Natural and artificial social learning
Social learning is powerful: agents that can learn from each other typically outperform similar agents who must go it alone. Across the animal kingdom, social learning takes many forms, ranging from emulation to gaze following to deliberate signalling. Human social cognition is particularly remarkable: unlike other animals, we query and correct each other, and work together to build a shared understanding of reality. But how exactly are we able to manage this, and why is it so helpful to distribute problem-solving among multiple agents? New research in artificial intelligence is generating insight into these questions, by developing algorithms which attempt to endow AI agents with social learning abilities, and studying what incentives can improve social abilities like cooperation in AI. This session looks at how social learning can help us build better AI and what insights we can gain from those AI systems about one of the most remarkable features of natural intelligence.
Speakers: Natasha Jaques, Sheila McIlraith (moderator), Jennifer Nagel
Digital constitutionalism and the futures of digital governance
The rise of the information society and the ubiquity of digital technologies in our lives brings new challenges for digital governance. Traditional institutions, processes, and even rights that were previously helpful in grappling with societal transformations might not be sufficient to address the variety of complex ethical and legal issues emerging from the development and use of these technologies, such as the emergence of technology corporations acting as private sovereigns. This talk explores the phenomenon of digital constitutionalism, in which various norms, laws, regulations and principles are now being articulated in order to limit the exercise of power—whatever the source—- and to balance those powers in the digital realm. In particular, I look at how an updated international human rights law can be a unique and important tool, no matter what the future of digital governance looks like.
Speakers: David Lie (moderator), Anna Su
Collective agency in evolution and AI
This session will focus on collective forms of agency through an integration of perspectives from multi-agent AI and theoretical evolutionary biology, in order to investigate the scope for reciprocal illumination between the disciplines of AI and evolutionary theory. When is a collection of agents a collective agent? What can the evolution of collective agency in natural agents teach us about the design of artificial agents, and what can the experience of designing artificial agents teach us about our own evolution? How does collective agency evolve, and how do the possibilities of collective agency shape and drive evolution in their turn?
Speakers: Kate Larson, Denis Walsh (moderator), Richard Watson
Building democratic social choice into recommender systems
Recommender systems are powerful, machine learning-based algorithms help us filter the digital landscape, deciding what content we see on our social media feeds, what movies and music are suggested to us, and what search results we encounter online. The recommender systems which drive social media platforms have been built through employing metrics of how users behave and engage online. What if users and/or public servants were given the opportunity to have a role in the design of these recommender systems? How could different methods of consultation and design—whether through expert consultations or citizen juries—improve the design of recommender systems. More broadly, how can we democratize the design of recommender systems?
Speakers: Peter Loewen (moderator), Taylor Owen, Jonathan Stray